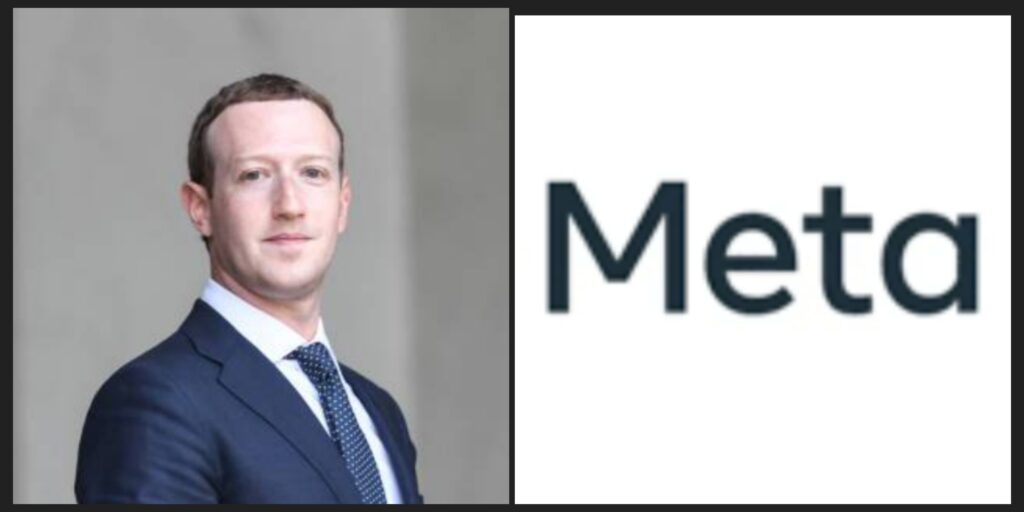
Source – Facebook’s hate-speech detection algorithms was found by company researchers to have under-reported less common but more harmful content that was more often experienced by minority groups such as Black, Muslim, LGBTQ, and Jewish users.
Platforms like Facebook, YouTube, and Twitter are banking on developing artificial intelligence technology to help stop the spread of hateful speech on their networks.
The idea is that complex algorithms that use natural language processing will flag racist or violent speech faster and better than human beings possibly can.
Doing this effectively is more urgent than ever in light of recent mass shootings and violence linked to hate speech online.
But two new studies show that AI trained to identify hate speech may actually end up amplifying racial bias.
In one study, researchers found that leading AI models for processing hate speech were one-and-a-half times more likely to flag tweets as offensive or hateful when they were written by African Americans, and 2.2 times more likely to flag tweets written in African American English (which is commonly spoken by black people in the US).
Another study found similar widespread evidence of racial bias against black speech in five widely used academic data sets for studying hate speech that totaled around 155,800 Twitter posts.
Facebook’s failure to check political extremism, [15] willful disinformation, [39] and conspiracy theory [43] has been well-publicized, especially as these unseemly elements have penetrated mainstream politics and manifested as deadly, real-world violence.
So it naturally raised more than a few eyebrows when Facebook’s Chief AI Scientist Yann LeCun tweeted his concern [32] over the role of right-wing personalities in downplaying the severity of the COVID-19 pandemic.
Critics were quick to point out [29] that Facebook has profited handsomely from exactly this brand of disinformation.
Consistent with Facebook’s recent history on such matters, LeCun was both defiant and unconvincing.